May 17, 2020
Preparation, Evaluation, and Selection of Informatics Platforms for Startups, Small Biotechnology Companies
By
John F. Conway and Sharang Phatak
Startups / early-stage biotech's are primarily focused on advancing scientific milestones. They may externalize much of their research and development which includes the data generation capabilities or start off with some basic laboratory setup with goals of eventually hiring researchers and expanding laboratory capabilities. Regardless of the operational model, research data is at the latest, generated on day one, and in many cases, such data exists even before the company is officially launched. At this stage, these research data are analyzed and exchanged primarily using commonly used office productivity software. It is from this point that research informatics falls behind the curve of actual data generation and impedes success. Herein we outline strategies and factors to drive the selection process of a logical informatics platform. The overarching goal is to help empower researchers to successfully traverse the data, information, and knowledge pyramid with the right technological solution(s). Our experience shows that if one follows these guidelines there is a greater chance of quickly arriving at data-driven decisions, innovate and help an organization to succeed.

Figure 1 Scientific Informatics Efficiency
1: Culture
Every scientist understands the importance of data and processes in their respective domains and is adept at deriving insights. However, the success of the overall organization is dependent on deriving insights across all research verticals. Permeating the thought process that data and processes are enterprise assets, should be free from silos but at the same time tagged with contextualized data across verticals is critical. This results in a decision-making process that is truly hypotheses/conjecture free or data-driven. An organization needs such a visionary or visioneer to establish this culture and get complete buy-in across the board from the management to bench folks before moving on to the next step of business requirements. All types of issues can affect alignment and company focus including but not limited to lack of effective business skills, founder syndrome, and poor strategy.
2: Business Requirements
As research data continues to be generated, exchanged via office productivity tools, emails, and shared drives for example biotech’s hit an inflection point when this approach becomes untenable. See Fig 1. With a multitude of scenarios possible, one at this point is the knee-jerk reaction to invite a few informatics vendors for product demonstrations or falling back on prior experiences and simply moving ahead with a known informatics platform. In the case of the former, multiple informatics vendors are invited without documenting actual business requirements and/or with a broad idea of what might be required. Such product demonstrations are unspecific and inefficient because:
A: Vendor applications scientists spend an inordinate amount of time trying to understand processes rather than highlighting features that might work for the stakeholder.
B: Stakeholders don’t necessarily get to visualize how their workflows would fit with a platform, might walk away with a limited idea on what vendors to evaluate and, at worse, disenchanted with the whole evaluation process.
In the case of the latter, what is critically overlooked, or many times assumed is that business requirements or workflows are transferable. It is also assumed that bench scientists will simply fall in line with a platform’s capabilities. This top-down approach is risky and might result in poor adoption. What works is a clear understanding at a highly granular level of workflows for each vertical e.g. chemistry, biology, pharmacology, ADME / PK, etc. These workflows should be categorized into the current (<1 year) to future state (>1 – <3 years) documented and discussed with vendors to drive a more focused product demonstration and a smoother evaluation process.
What good is an accurate data ingestion, if one cannot logically extract, visualize, analyze, and possibly integrate data? The requirements and business workflows beyond data ingestion should also be included. Data and processes are your family jewels and should be guarded accordingly. This also means one should be prepared to have very shareable and contextualized data to future proofs one’s near and distant future of expansion, acquisition, or merger and will positively drive the value of one’s company!
Working with the bench scientists and driving a consensus approach within a team, and the overall organization maximizes the probability of success of subsequent platform implementations. These detailed business and workflows requirements allow people and processes to drive technological decisions that are aligned with an overall organizational data and process strategy. This is a people business and scientists must be empowered to efficiently drive their research with the appropriate technology solutions.
3: Data and Data Analyses
Every organization generates data of myriad types. There is data derived from experiments (e.g. chemical structures, quantitative, qualitative), computations, modeling, and predictions, etc. There is data about data i.e. metadata (e.g. descriptive, process) and unstructured data (e.g. documents, images)
Understanding these data, data types, and data calculations are critical in the choice of platform(s) selection. It is routine to capture certain data types (e.g. chemistry, standard primary / secondary screening assays) in a relational database however, the complexity of these data types and/or calculations is only increasing. Any evaluation should prioritize the ability of platforms to natively (as much as possible) analyze such calculations. If not, the potential options to maximize automation by integrating specialized / custom analysis software with an informatics platform should be considered. In order to extend the usability of this data e.g. analyses in a translational discovery setting using ever-increasing genomics data, address reproducibility issues and deriving insights is possible by capturing appropriate metadata. The importance of metadata cannot be overstated especially when information is the biggest asset in this industry. Capturing appropriate metadata using standardize vocabularies and in machine-readable formats early in the process is advised and perhaps non-negotiable despite the seemingly additional burden. Doing so will lay a solid foundation to be FAIR data compliant and assist with a translational drug discovery approach.
This stage should result in a collection of data types, examples, etc. for platform evaluations. The choice of platform(s) should be amenable to capture all these data, data types, metadata, and analyses. Aligned with the right business requirements an informatics platform then should be able to present Model Quality Data (MQD) for mining, predictions, etc.
4: Technology
Cloud technologies are at the forefront of any discussion surrounding platform evaluations. Have a very well thought out set of reasons why your startup would not be an agnostic cloud-first company. We will not discuss the advantages of cloud technologies like adoption and lower support and maintenance costs, but instead provoke other thought processes around automatic data ingestion, integration, and transfer.
As research data generation processes mature, biotech companies often seek to maximize the usage of any given informatics platform by means such as automation or simply seek a lab of the future. It is also natural to see a more complex informatics landscape e.g. specialized informatics tools, modeling capabilities, etc. which inadvertently result in unexpected data silos or data types incompatible with informatics platform capabilities. There are multiple ways to address this issue, but at the very least setting up automated processes to integrate as much of data and types into a platform of choice minimizes the severity of issues related to data silos. These custom processes need supporting technologies for implementation e.g. development framework, computing platforms. , If business requirements warrant additional reporting, analytics, dashboarding capabilities that span beyond one’s informatics platform of choice it is advisable to investigate enterprise-level data management options such as data warehouses, data lakes. Doing so will ensure organization-wide informatics requirements are met.
As we mentioned before, a clear understanding of culture, business data, and processes make it easier to envision such use cases and incorporate them in a pre-defined scoring metric for technology platform evaluations.
5: Evaluation
So, by now we have established a data/process-driven culture, understood business requirements, data types and aligned technology needs to an organization's growth road map. This results in a firm set of criteria to evaluate vendor platforms. Every evaluation should have a clear and realistic objective, time frame, set deliverable, and finally, a score based on platforms existing and future capabilities. It is helpful to invite a few different vendors for product demonstrations, to determine at a high level whether one platform or a minimal set of platforms will serve as a minimum viable product (MVP) for an organization’s informatics need. It is critical to stick to the requirements and metrics obtained from the step above and have a complete focus on extracting the maximum from an evaluation for one's organization. It is quite easy to get distracted on “nice to have” when “must-have” should be the goal. What is “nice to have” must match the future state workflows as defined in Step 1 or at least the vendor should be able to present road maps that align closely with one's requirements. At this time, you also get to see the professionalism of a scientific software company. Their culture is critical as well and should be part of the decision criteria when selecting an informatics partner! You do not want to partner with a boat anchor!
There is currently a plethora of companies that have “tools” that you can get for free (e.g. CDKit, EPAM, Knime, PostgreSQL, Python, R, RDKit, etc.), or for a cost. Building your own tools is not recommended unless you are doing something completely novel and not handled by any software or technology, which in our experience is usually very unlikely. The top companies out there that the major biotech’s and biopharmaceuticals are using are: Benchling, Biovia, ChemAxon, Collaborative Drug Discovery, Delta-Soft, Dotmatics, Genedata, IDBS, Sapio Sciences, and Scigilian, etc.
Assuming the right culture is set in step 1, the team should be motivated considering by this time they are deep in to running experiments and generating results. This is the most important factor for any successful evaluation exercise and often require a good supporting cast to drive this process.
6: Personnel
Smaller biotech’s usually start with one or two full-time informatics resources. They are supported by consultants (independent or consulting firms) and/or contractors. Consultants possess extensive professional/deep domain knowledge and offer an expert opinion based on client needs. They may or may not take on technical solutions delivery. Contractors deliver against specific tasks. This distinction must be clear. Regardless, in addition to domain knowledge and/or technical skills they should possess good people skills, e.g. empathy, trust, critical thinking ability to successfully deliver in a fast-paced environment. Seek recommendations and ensure you are not working with someone who is biased for any set of reasons.
The above qualities are also key requirements for an effective leader. Leadership is one of the most important parts of this and an organization’s culture. Often strong leadership, a champion, is the key differentiator between success and failure. Weak leadership will result in a failed endeavor despite heavy investments in people and technology. We have lived it from multiple professional personas. All of this described has to take into account the WIIFT (What’s In It For Them) simplify processes, enhance collaboration, discovery, and very importantly replication and repeatability!!
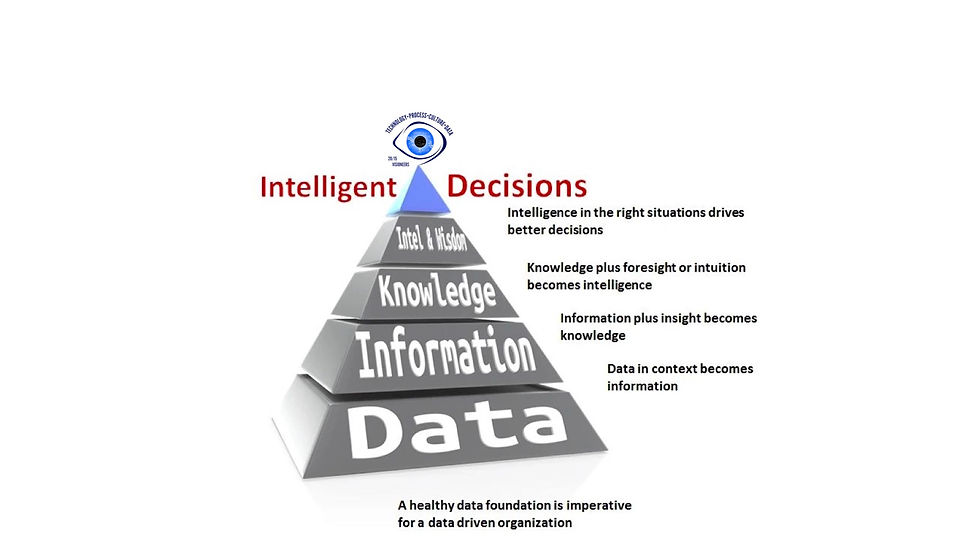
Figure 2 Intelligence Pyramid- Data and Processes
Biographies
John is the Founder and Chief Visioneer Officer at 20/15 Visioneers LLC. He has 30 years of experience in sciences, materials, chemical, and energy verticals. For both biotech and large organizations. Previous leadership roles include Global Head of R&D IT, AstraZeneca, Global Head of R&D Innovation and Thought Leadership, Accenture, Head of R&D Strategy and Solutions, LabAnswer, Vice President of Professional Services and Informatics, Schrodinger, Sr. Director of Professional Services and Solutions, Accelrys(Biovia), Global Director of Discovery Informatics, GSK, Computational Methods Developer, Cheminformatics and Bioinformatics, Merck and Company Inc.
Sharang is a research informatics professional with a background in computational drug discovery. He has extensive expertise in strategy consulting, technical design, development, and delivery of scientific informatics solutions in the pre-clinical research space for small, large pharmaceutical companies and research organizations. He is the owner of EmpowerSci Informatics LLC and consults for multiple small-large biotechnology companies in Boston. He earned his Ph.D. in Biomedical Informatics from The University of Texas Health Science Center and a post-doctoral fellowship at Vanderbilt University. His predominantly client-facing work experience spans scientific / research informatics product companies, large non-profit drug discovery centers, and consulting companies. He can be reached at sharang@empowersci.com
Comentarios