January 28, 2020
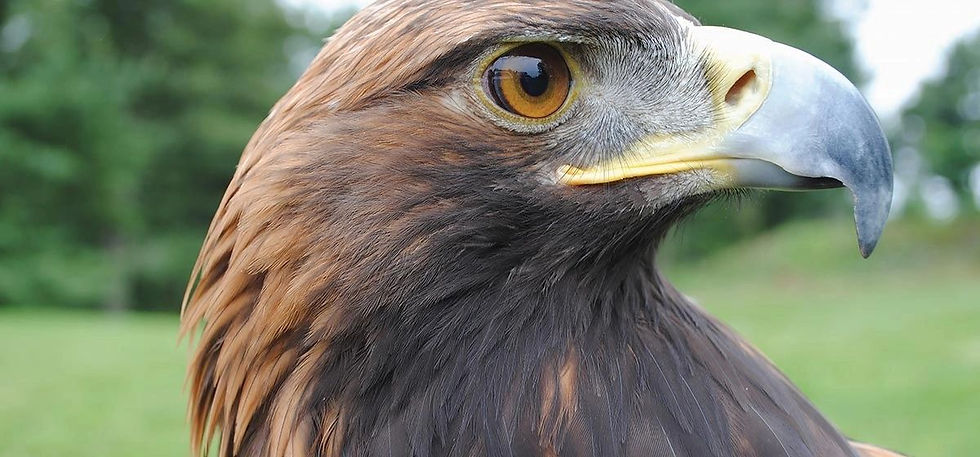
By John F. Conway
Data and process-driven R&D organizations collect many different types of information. Their data environment is usually diverse and expansive, and most times, not FAIR (Findable, accessible, interoperable, and reusable). Never underestimate the health and shape of a client’s data environment. What comes along with that, is never underestimate the health and shape of a client’s process environment. Greater than 80% of all data migration projects fail because of data quality, time and budget overrun and poor scoping.
Obviously, there are different types of scientific data assessments and they can range from simple cataloging to assessing FAIRness and the value of migration. What comes with migration sometimes includes a process of ETL (extract, transform and load) where the transform part is data cleansing, fixing, duplicate checking and even adding additional contextualization.
It's critical that the level of detail being asked or offered is captured, concise and clear. Like any statement of work, sometimes people assume what the other is saying or describing, so any concrete examples will help with the alignment of objectives and goals.
Major areas of concern or assessment are: Security Authentication FAIRness Authorization Proper metadata/contextualization Level of Proprietariness Technology Technology version(s) Integrity Retention Policies Quality Confidence
There are 4 steps to running an assessment. They are Inventory, Classification, Actual Audit and Records retention compliance, and Reassessment. We have done this for others and we can do this for you!
Comments